Wind turbines extract kinetic energy from the atmospheric boundary layer (ABL), the lowest region of the atmosphere, which is characterized by a wide range of variability due to turbulence, stratification, complex terrain, and wakes from nearby wind turbines. However, current prediction models do not account for the effects of this variability resulting in significant errors in wind power forecast. Our research involves developing models to predict energy generation from wind farms for the range of atmospheric conditions. Wind plant operators and balancing authorities use forecasts to optimize integration of renewable wind power and delivery to the electric grid. Analogously, we investigate turbulent flow and turbine configurations for river and tidal turbines. We can use our improved understanding of feedback between turbines and flow in turbine arrays under variable environmental conditions to develop models to optimize renewable power generation.
Development of improved wind power forecast models for wind farms in complex terrain
We are developing improved models for energy generation assimilating turbulence measurements from tall towers and nacelle-mounted wind LiDARs under realistic field conditions to evaluate the performance of the models compared with SCADA data from utility-scale wind turbines. Measurements of wind speed and direction, turbulent fluxes, wind shear, wind veer, yaw error, temperature, pressure, and relative humidity are used to improve power predictions for grid integration. Results from our research are reported in Carbajo Fuertes, Markfort, and Porté-Agel (2018) and Vahidzadeh and Markfort (2019).
- Complex terrain and density stratification affect wind power plant performance and make prediction of wind energy particularly challenging.
- Turbulence enhances supply of energy to wind turbines, but also leads to fatigue and premature failure of turbine components.
- We utilize measurements using Doppler wind LiDAR and meteorological towers, as well as wind tunnel studies, to improve models of environmental flows and wind turbine wakes.
- Simulations of the interactions between wind turbines and the atmospheric boundary layer can be used to optimize wind power plant design and control to maximize power production based on local conditions.
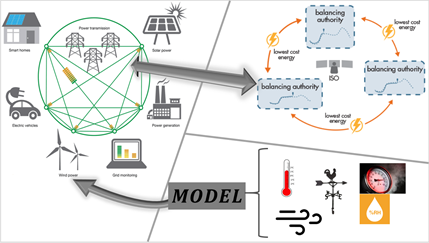
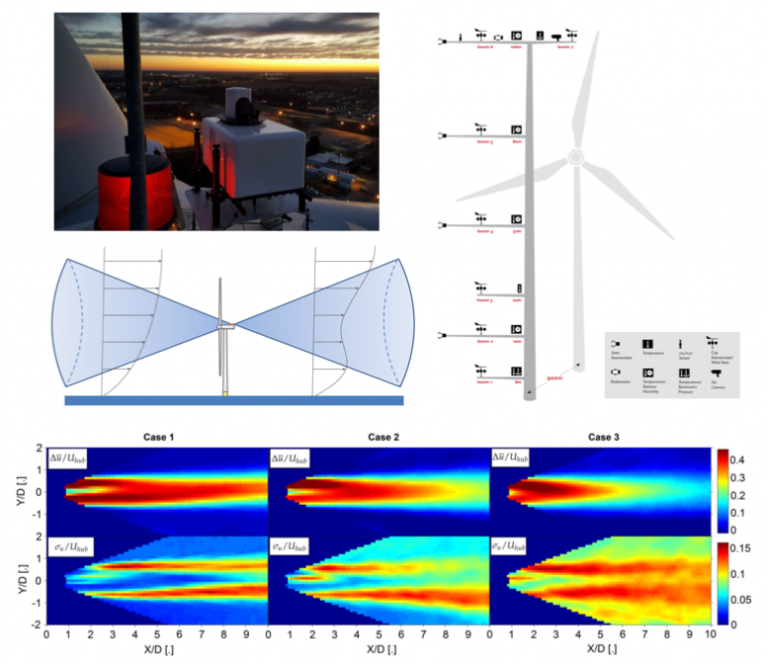
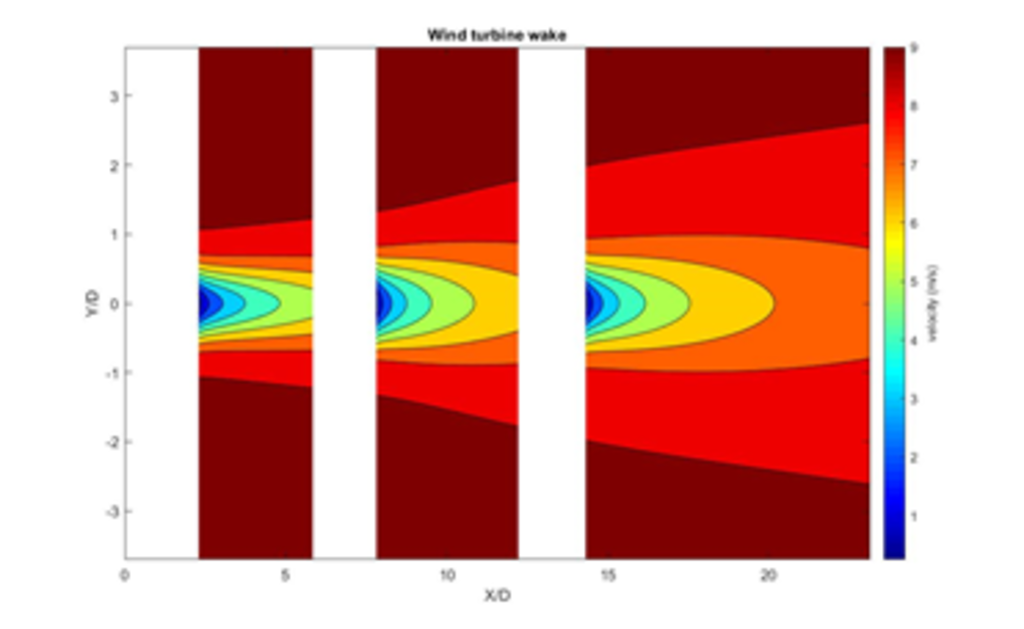
Wind farm optimization using an analytical wake model with performance data from wind turbines
Wind turbine wakes significantly affect wind farm power generation. Reducing the wake effect can significantly increase the power generation and reduce turbine fatigue. Our goal is to develop a procedure that wind farm operator can easily utilize with existing SCADA system to reduce wake effect. The procedure employs wind turbine power curve modeling and incoming wind speed correction to account for high turbulence intensity. The analytical wake model is augmented with on-line SCADA data. The model can help to optimize wind farm performance as well as improve understanding and mitigate impacts of low wind regions on risks to wildlife such as bats.
Evaluating the effect of flow depth on wake recovery of hydrokinetic turbines
In-stream hydrokinetic turbines have the potential to produce a significant amount of clean energy from river and tidal currents. We are investigating the effects of flow depth and turbine spacing on the performance and wake behavior downstream of hydrokinetic turbines. Using Laser Doppler Velocimetry, we measured the mean velocity and turbulence in the wake under varying flow depth conditions and found that for equivalent inflow conditions, a deeper flow resulted in more rapid wake recovery (Aghsaee and Markfort, 2018).
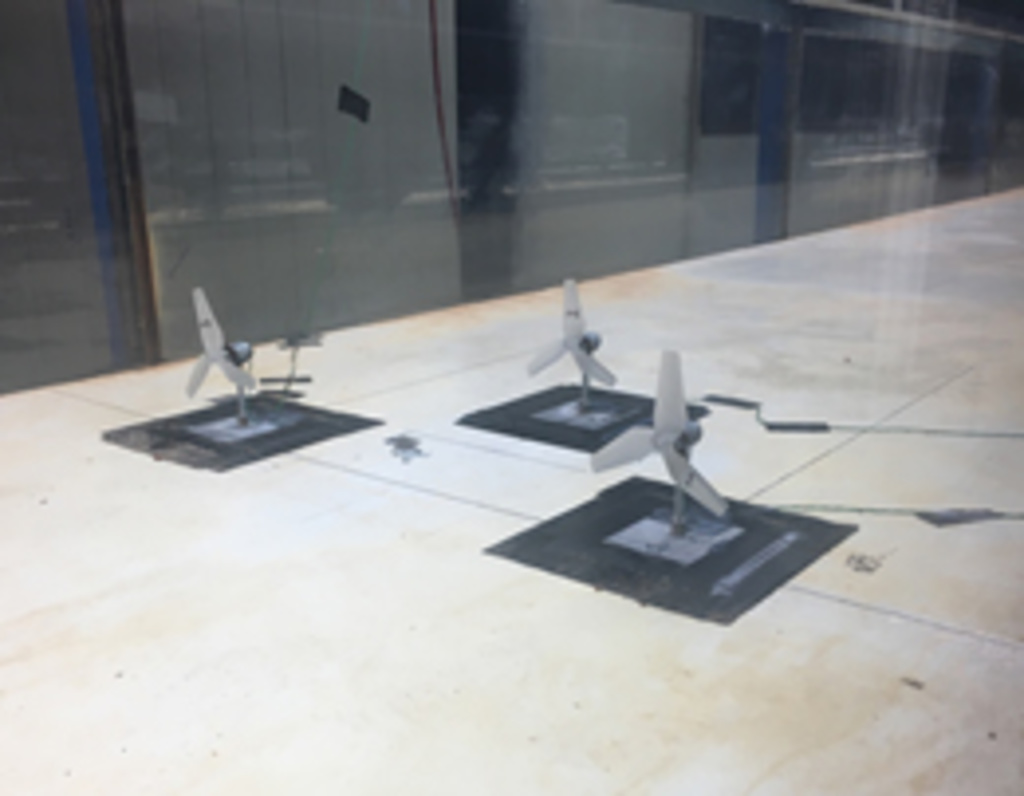
Team members and collaborators: Mohsen Vahidzadeh, Jian Teng, Payham Aghsaee, Peter Brugger (EPFL), Fernando Carbajo Fuertes (EPFL), Fernando Porté-Agel (EPFL)